Artificial Intelligence face will be accurately identified by AI
by Ready For AI · Published · Updated
In the future world, it will be difficult to tell whether this is an artificial intelligence face or a real face. Maybe we can only rely on artificial intelligence to identify it. Does this sound ironic?
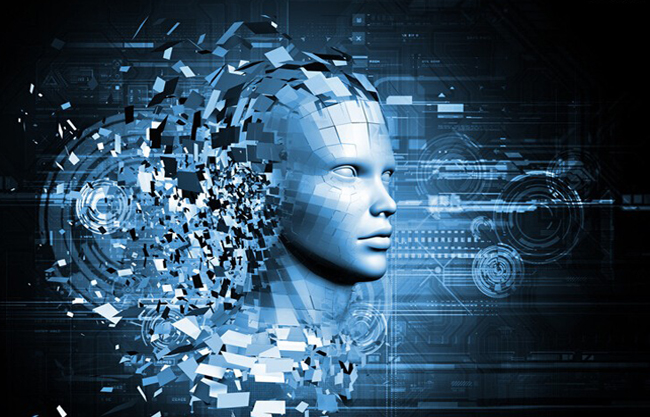
What is an artificial intelligence face?
Artificial intelligence face refers to the dynamic face synthesized by artificial intelligence technology, including the realization of facial expressions and movements. Its true level is often beyond your imagination, especially the popular application Face Swap. Let’s take a look at some projects similar to this technology:
- Deepfake
Deepfake, the combination of “Deep learning” and “Fake”, is a deep-based character image synthesis technology that combines and overlays any existing image and video onto source images and video.
Deepfake allows people to create fake porn videos, fake news, malicious content, and more with simple video and open source code. Later, Deepfak also launched a desktop application called Fake APP, which allows users to easily create and share face-changing videos, reducing the threshold for many ordinary users to use.
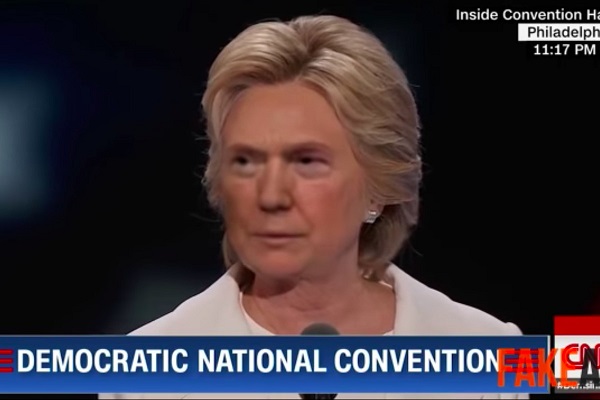
Trump’s face was transferred to Hillary.
- Face2Face
Face2Face is also a “face-changing” technology that has caused great controversy. It appeared earlier than Deepfake and was released at CVPR 2016 by the team of scientist Justus Thies of the University of Nuremberg, Germany. This technology can realistically copy a person’s facial expressions, facial muscle changes, mouth shape, etc. in real time to another person’s face. Its effect is as follows:
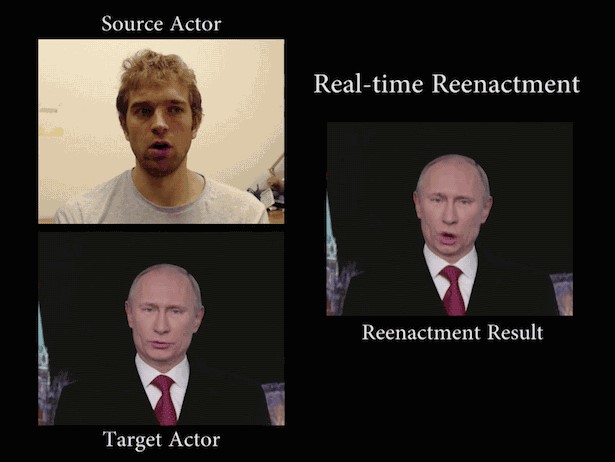
- HeadOn
HeadOn can be said to be an upgraded version of Face2Face, created by the team of the original Face2Face. The research team’s work on Face2Face provides a framework for most of HeadOn’s capabilities, but Face2Face can only convert facial expressions, and HeadOn increases the movement of body movements and head movements.
That is to say, HeadOn can not only “change face”, but also “change people” – according to the action of the input character, change the facial expression, eye movement and body movement of the character in the video in real time, so that the person in the image looks like It is really the same as talking and moving.
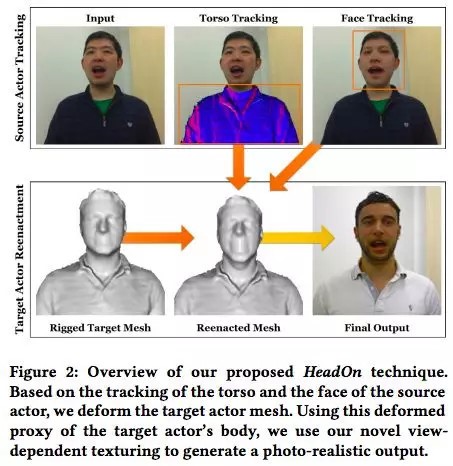
In the paper, the researchers called the system a “real-time source-to-target replay method for the first human portrait video, which enabled the migration of trunk movements, head movements, facial expressions, and gaze gaze.”
- Deep Video Portraits
Deep Video Portraits, a paper presented to researchers at Stanford University and the Technical University of Munich, to SIGGRAPH 2018, describes an improved “face-changing” technique that reproduces another face in a video with one person’s face. Departmental movements, facial expressions and talking patterns.
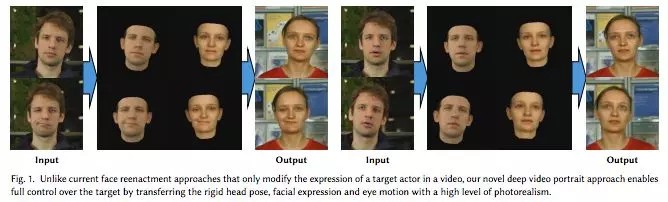
- paGAN
The latest “face-changing” technology that has caused great repercussions comes from the team of Chinese-American professor Li Hao, who developed a new machine learning technology, paGAN, which can track faces at a rate of 1000 frames per second, using a single photo. Real-time generation of ultra-realistic animated portraits, the paper has been received by SIGGRAPH 2018.
This 3D face generates a corresponding expression through the action of the person in the middle.
What is the anti-AI face change?
The US Department of Defense has developed the world’s first “anti-AI face change detection tool” designed to detect AI face change / face change fraud technology. Nowadays, the AI face-changing technique represented by GAN is popular, and the corresponding face detection and recognition technology has to be upgraded. This is just the beginning of a long and wonderful AI arms race.
Detecting the authenticity of digitized content usually involves three steps:
- The first is to check if there are two images or signs of video stitching together in the digital file;
- Second, check physical properties such as the illuminance of the image to find signs of possible problems;
- Finally, check if there is a logical contradiction in the image or video content.
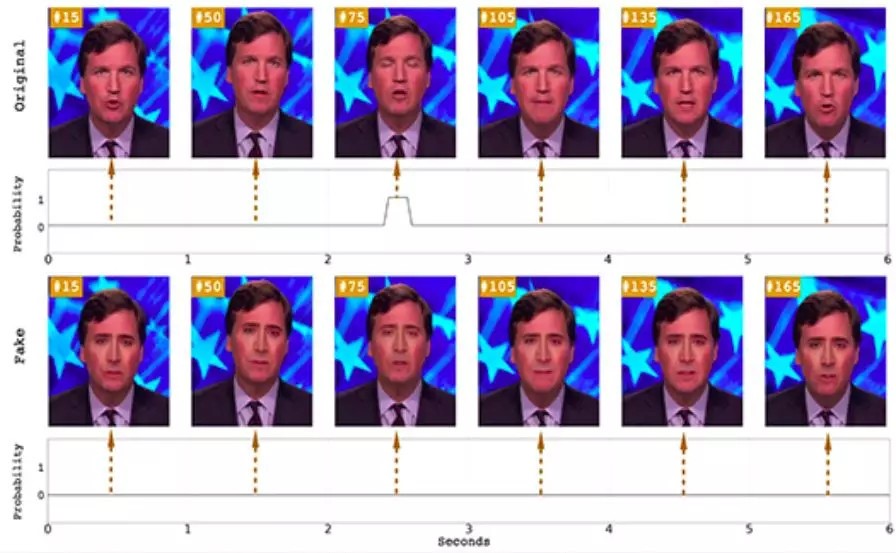
DARPA’s MediFor project brings together world-class researchers to develop technologies that automatically assess the integrity of images or video and integrate them into an end-to-end platform. If the project is successful, the MediFor platform will be able to automatically detect changes to the image or video and give detailed information on how it was changed and the reasons for determining whether the video is complete, in order to decide whether suspicious images or videos can be used. Used as evidence.
DARPA’s tool is based on the joint discovery of Siwei Lyu, a professor at the State University of New York at Albany, and his students, Yuezun Li and Ming-Ching Chang, which are false faces generated by AI technology (generally known as DeepFake), with little or no They will blink because they are trained using blinking photos.
They combined two neural networks to more effectively reveal which videos are AI-synthesized. These videos tend to ignore spontaneous, unconscious physical activities such as breathing, pulse, and eye movements. By effectively predicting the state of the eye, the accuracy rate is 99%.
AI's challenge against AI
At present, there is still a lack of wide applicability for tools or applications that distinguish a large number of authentic and indistinguishable digital content. The scalability and robustness of such tools in relational applications such as forensics, evidence analysis and authentication. Sex can not fully meet the needs of practice.
David Gunning, head of DARPA’s project, said: “In theory, if you use all the techniques at this stage to detect the false results generated by GAN, you can learn to circumvent these detection techniques.
Hany Farid, a digital forensics expert at Dartmouth University, said that the current AI anti-face tool mainly uses such physiological signals, at least so far these signals are difficult to imitate. The bringing of these AI criminal investigation and evidence collection tools only marks the beginning of the AI arms race between AI video counterfeiters and digital criminal investigators.
According to Hany Farid, one of the key issues at the moment is that machine learning systems can accept more advanced training and then go beyond current anti-face tools.
DARPA researchers say the agency will continue to conduct more tests to “ensure that the identification technology under development can detect the latest fraud technology.”