The status and problems of Machine Learning crime prediction
by Ready For AI · August 23, 2018
The use of machine learning crime prediction has become a hot technology, and real-time detection of "criminal behavior" in the crowd has become a reality.
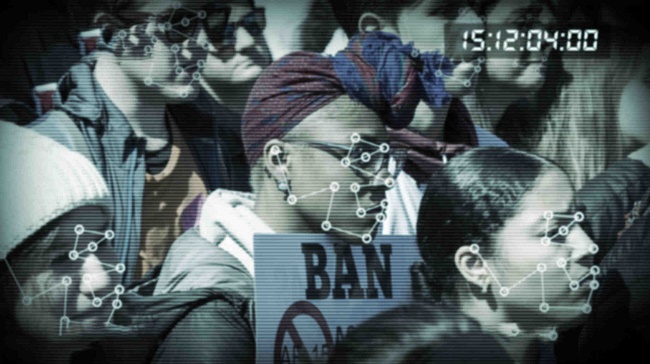
Machine learning crime prediction application
This machine learning system which can detect “criminal behavior” was developed by researchers at the University of Cambridge, the National Institute of Technology of India and the Indian Institute of Science and Technology. It is a system with artificial intelligence technology that uses a hovering quadcopter and uses the camera to detect the body movements of everyone.
Then, when the system recognizes the aggressive behavior, such as punching, kicking, assassination, shooting, strangle the neck, etc., it will issue an alert with an accuracy rate of 85%. But it does not currently recognize faces, it just monitors possible violent behaviors between people.
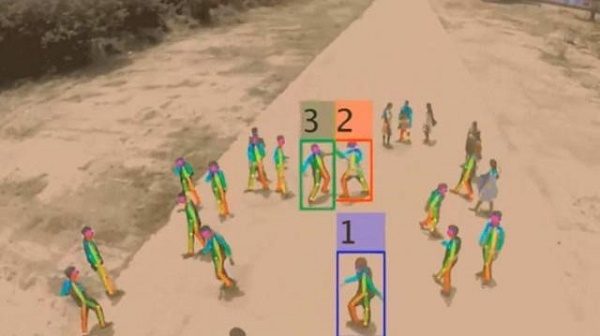
The system can be extended to automatically identify people who cross the border illegally or to alert when public places find kidnappings and other violent acts. Therefore, in theory, this drone with the machine learning crime prediction technology can help the police to combat crimes or help soldiers find enemies among people.
The status of machine learning crime prediction
When AI detects violent movements such as killing, punching, kicking, shooting, and stabbing, the system first uses Feature Pyramid Network(FPN) to detect all human beings in the image and marked their 14 important parts including the head, upper limbs, and lower limbs. Then it will draw the overall frame of the human body, use the SHDL network to analyze data such as the orientation of the limbs and determine whether those people are using violence to commit crimes.
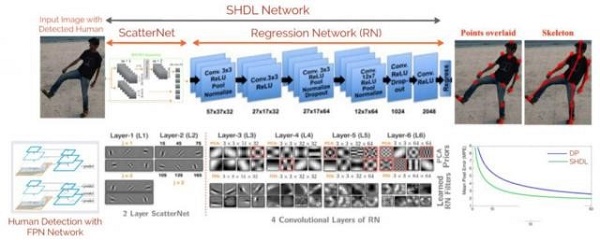
Of course, the effect of AI crime prediction is also very much related to the amount of data that needs to be processed. In general, the accuracy rate will be higher when the number of people the system needs to handle is small. When only one person’s data needs processed, the system accuracy rate can reach 94.1%. If the number of people exceeds ten, the accuracy rate will drop to 79.8%.
Similarly, the machine learning crime prediction application also has a difference in accuracy when detecting five types of violence, it does not recognize these actions very accurately such as killing, punching, kicking, shooting, and stabbing.
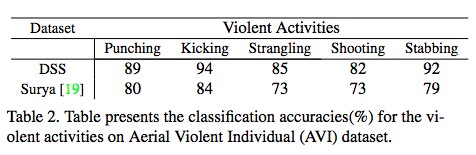
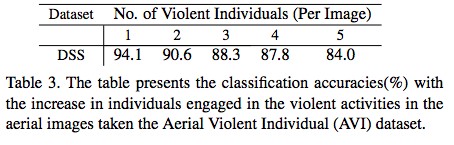
On the other hand, there also are certain restrictions on the use conditions of the drone itself. In the real application scenario, the drone can’t be directly close to the criminals and only the high-altitude monitoring can be implemented, which reduces the visibility and detection accuracy to some extent.
User information leaks that cause flooding should be avoided
2017 is a year of extremely data breaches, according to a recent report by Gemalto “2017 Poor International Security Practices Take a Toll”, the data stolen in the first half of 2017 alone exceeded the total amount of stolen data for the whole year of 2016.
From January to June 2017, an average of 10.5 million records were stolen every day. Many data breaches were caused by external hacking attacks, the resulting records were stolen or lost accounting for 13%. In contrast, data breaches due to internal malicious disclosure, employee negligence and unintentional disclosure accounted for 86% of the stolen data.
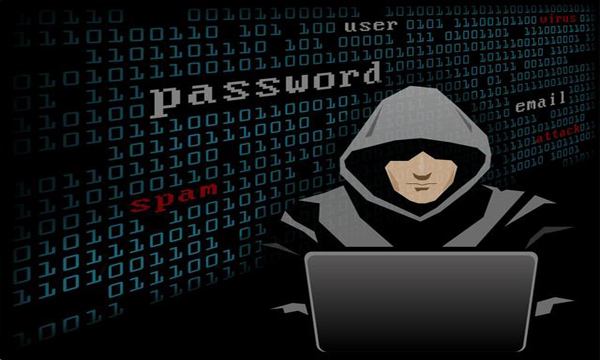
The previous Cambridge Analytica scandal caused an information leak of 87 million people and affected the results of 2016 Donald Trump’s participation in the US presidential campaign. The Facebook data breach incident once again showed people the danger of information leakage, “Being behavior” or “inducing behavior” became a bad operation for many data companies to use user information.
It is conceivable that if the machine learning crime prediction system is used maliciously, the risks are even more serious. Like the facial recognition technologies, such as Amazon Rekognition service, have been adopted by the US police, and these systems are often plagued by high false positive rates or are simply inaccurate, so such a technology may take some time to be combined with drones to accurately predict crime.
The object being monitored needs “right to know”
In the process of AI monitoring, “informed” has indeed become the focus of an ethical debate, there are constant disputes about “how to monitor” and “whether or not to know”. It is true that with the continuous development of behavior recognition technology, many recognition technologies can achieve “uninformed”: that is, the subject does not need to cooperate, and can still achieve accurate recognition.
This undoubtedly brings artificial intelligence ethics to a new level, the information is no longer owned by me, and it is really worrying. In the field of police and criminal investigation, AI monitoring is still convincing. But in other civil and even commercial fields, how to balance the “acquisition of information” with the guarantee and meet the basic right of the monitored object will become an important question.
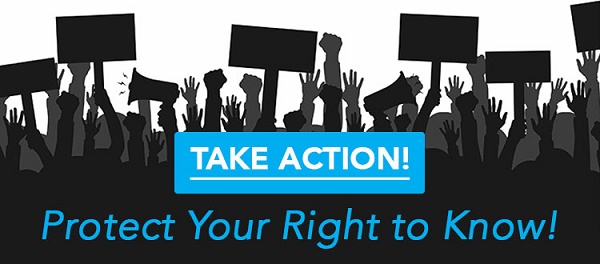
Obviously, many large companies are actively seeking a balance between such “acquisition of information” and “protection of privacy”. Facebook Chief Operating Officer Sheryl Sandberg announced that in response to the EU’s new privacy regulations, Facebook will make it easier for its 2 billion users to manage their personal data.
The General Data Protection Regulation (GDPR) implemented in the European Union is the largest revision of personal privacy data regulations since the birth of the Internet. The purpose of the regulation is to give residents in EU member states more authority to manage their own information and to stipulate how companies to use user data.
Conclusion
The emergence of machine learning crime prediction technology provides a useful aid for the security and criminal investigation fields. However, as an area that is extremely sensitive and influential, the issue of predicting accuracy is still not to be ignored, after all it is never a good thing to wrong a innocent person.