One of the directions of Chinese Artificial Intelligence research: Neurocognitive
by Ready For AI · October 2, 2018
If you disagree with the direction of AI research based on data science, then you can look at the Chinese artificial intelligence research direction.
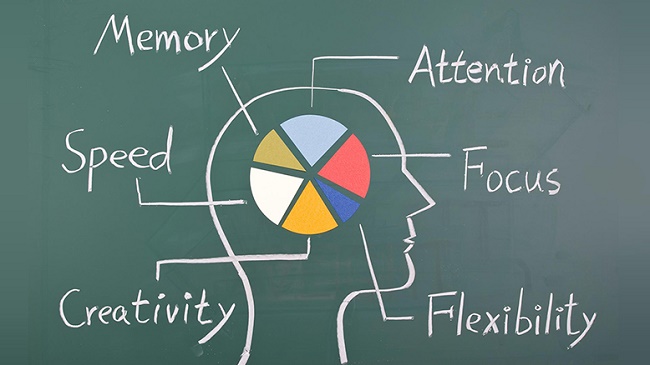
Why use the method of neurocognitive?
The traditional approach is to use the world model and the machine model to achieve intelligence, which means that we need to know all the details in the two models, and then get a engineered solution. For example, in early industrial robots, we knew the dimensions of its joints and arms, and then used statistical methods to calculate its path of operation to obtain a series of mathematical solutions. However, this research team from China found that this solution is only suitable for automotive assembly work where the production environment is relatively simple. If we let the robots work in the 3C field, they are not well done. Because the environment facing robots is constantly changing, developers cannot use a single world model to describe the complex environment in which robots are located.
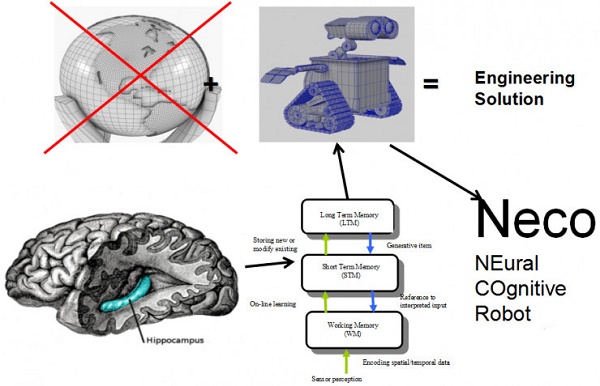
This is the structure of the future robots envisioned by the team. They are digitally modeled according to the cognitive principles of the brain and call it the “memory model”. The team’s researchers believe that the most important reason for human intelligence is the change in the number of memory. So they tried to apply this model to the robot, which is to install a “machine brain” that can be used for memory. As a result, engineers no longer need to build complex world models for robots, but the robots themselves build them. They call them NEural COgnitive Robots.
At the same time, the team believes that only the “machine brain” of memory function is not enough. It also requires a lot of sensors to work together so that the “machine brain” can get enough external information, then process it through the neural network, and finally output it to the execution module. This is a cyclical process consisting of judgment, feedback, decision-making, and execution. It is also the process that researchers believe can produce intelligence.
Research content on the direction of neurocognitive
The team’s core researchers believe that the development of robotics to the present, relying on a separate algorithm can not meet their expectations of the level of robot intelligence. Even if engineers and code are constantly stacked, the level of intelligence in robot performance is very low. They believe that neurocognitive methods have the potential to fundamentally improve the intelligence level of robots.
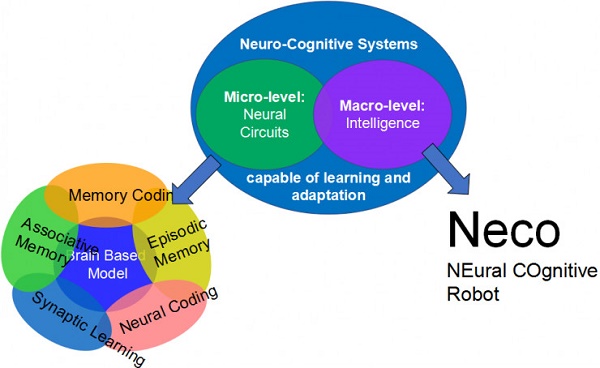
The team’s main research direction is to simulate some basic rules of the nervous system, such as: Simulating the working principle of Synapse. They think that the number of this neuron model reaches a certain level, the system may show the level of intelligence it should have. These large numbers of neuron models will be classified according to cognitive psychology, including memory coding, associative memory, synaptic learning, neural coding, and episodic memory.
It is hoped that the number of neurons will increase to change the level of intelligence of the robot, which means that they do not care whether the neuron model is close to the human biological system. They emphasize the ability of robots to adapt to the environment is the true level of intelligence of robots.
The core of the "machine brain": Brain-like chips
The team’s first experimental project was to use robots instead of experimental mice. They simulated an experiment done by Edward Tolman in 1940: let the mice find a piece of cheese in the cross maze, but they replaced the mouse with a robot, let the robot to find the cheese in different environments based on the landmarks.
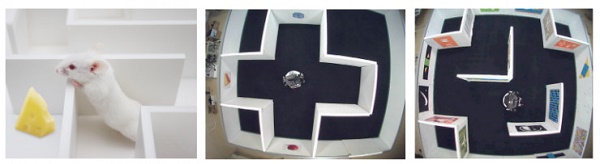
In order to solve this problem, the team members designed a brain-like chip architecture to realize spatial perception and visual processing. In this experiment, space just has only four directions, and the robot needs to be able to identify some objects. They use the overall image as input and create a corresponding memory representation for it, then enhance it through neural connections and finally output the corresponding results. The robot selects a neuron in the four neurons of the system and moves in the direction it represents.
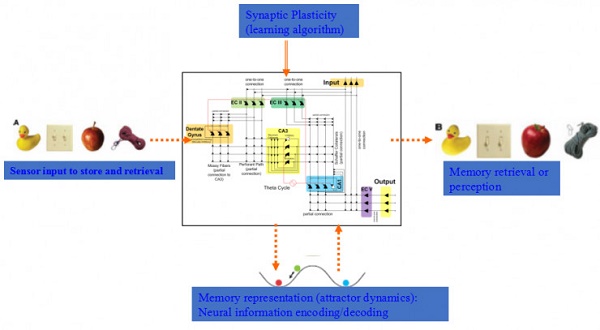
Most of the architecture uses neurocognitive models, but there are still some places where robotic engineering methods are used. The team said that when the neurocognitive model is mature, this part can be replaced.
Introduction to some implementation principles
The team provided a PowerPoint document to introduce the techniques and principles used, and it is also here for your reference.
- Brain-Inspired Neural Circuits
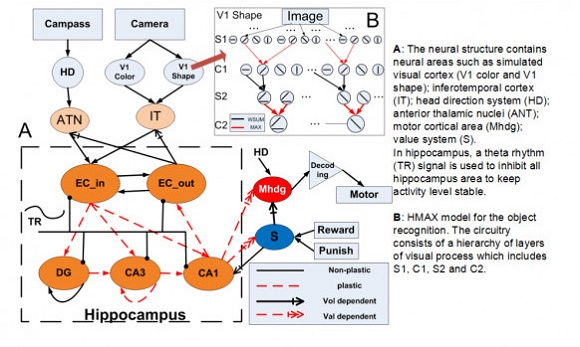
- HMAX vision system
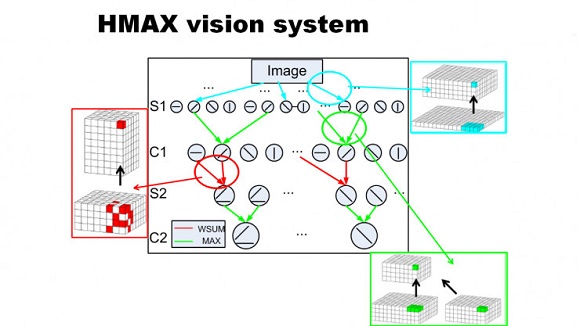
- Episode memory model: Recurrent Synapses
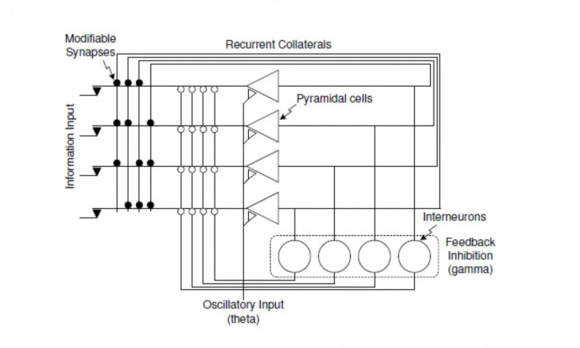
- Spatial cognitive memory model: RatSLAM
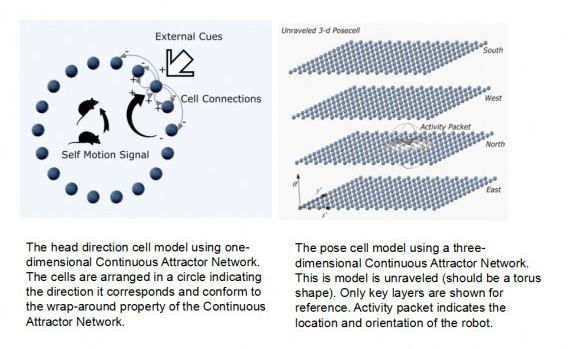
- Build global map
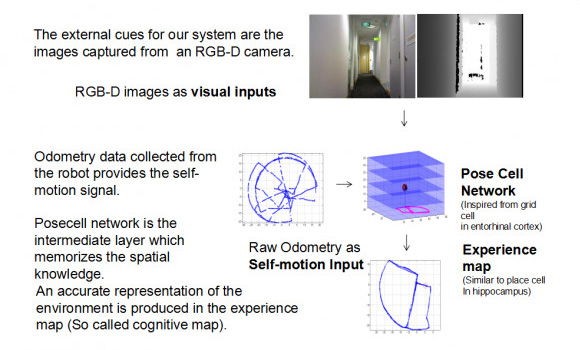
- Cerebellar model for controlling differential movement
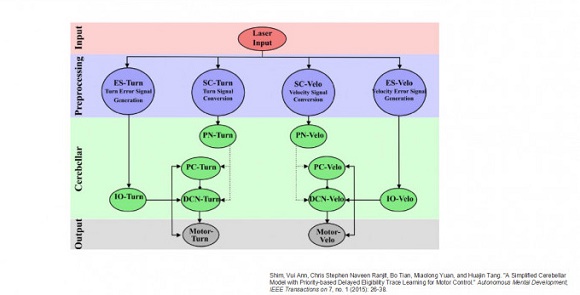
- Navigation experiment
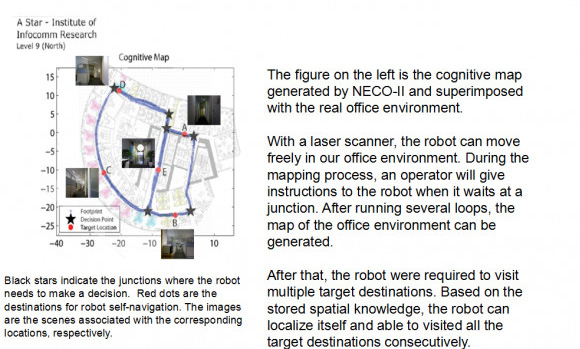
- Cognitive map algorithm
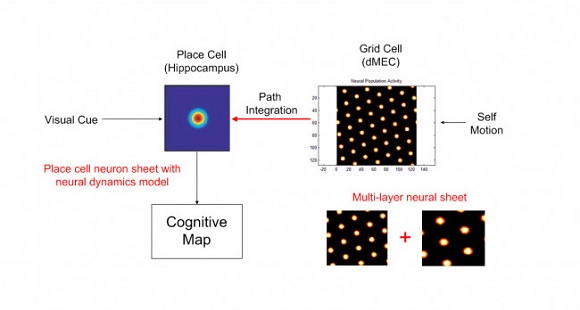
- Spatial cognitive map generation
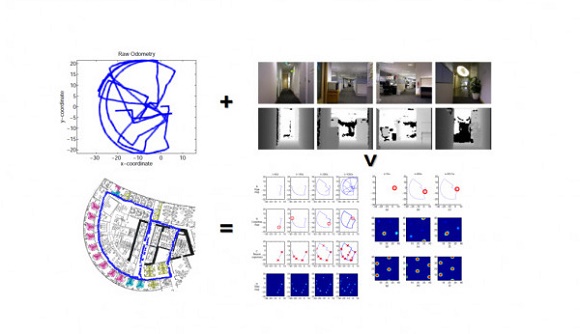
- Actual effect and neuron activation position
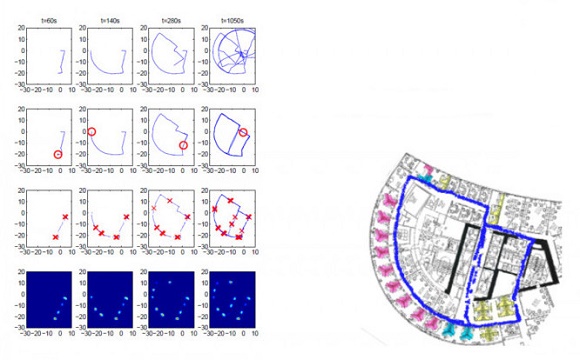
- Overall model of spatial cognition and task
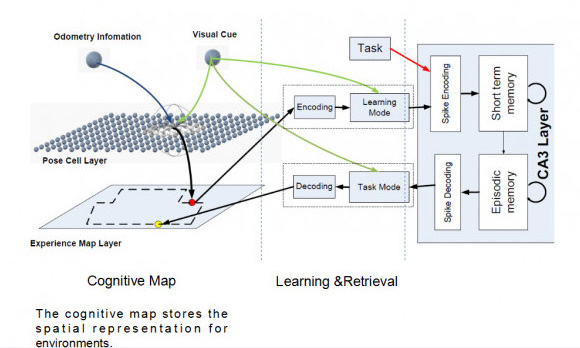