6 typical examples of Artificial Intelligence in healthcare
by Ready For AI · Published · Updated
Here are 6 typical examples of artificial intelligence in healthcare, proved that some technologies have accelerated the use of artificial intelligence.
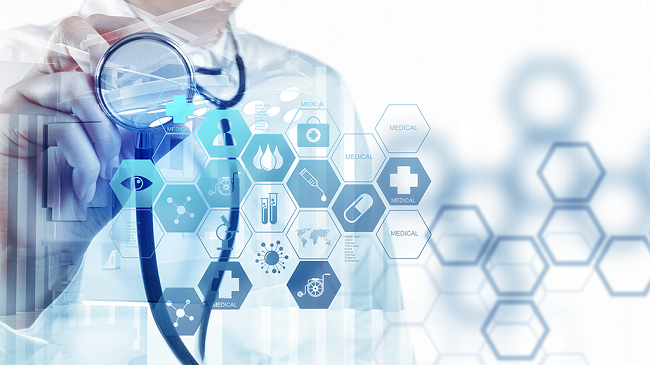
1. Big data and flu prediction
As early as 2008, Google has launched a flu prediction service: by detecting the user’s search on Google, it can effectively track the signs of the flu outbreak. For example, the number of searches for keywords such as “headache fever”, “disgusting” and “sneezing” is about 200,000 times a day in a certain area. When a certain period of time, the number of searches for these keywords rises sharply to 600,000-800,000, Google server will judge the need to pre-judgment and alert. Google will also correlate users’ search results by analyzing users’ emails to more accurately determine the occurrence of such outbreaks. In addition, Google Baseline Study hopes to build a huge human health database to find a fully healthy human genetic model. According to this database, as long as the user’s health data is found to be different from the model, Google will remind the user of possible health problems and prevent it.
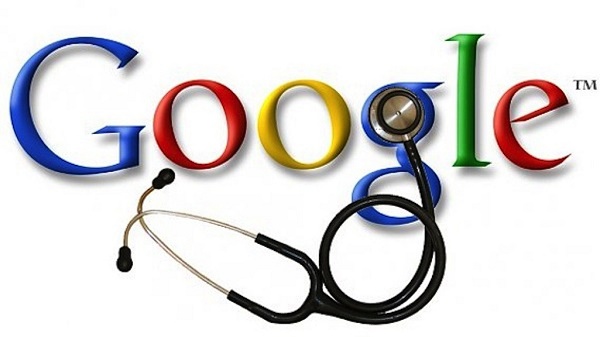
The Google Fit platform has developed a range of wearable devices, including clothing, shoes, bracelets, glasses, and more. These products are constantly collecting massive amounts of biometric data and combining them with Google’s baseline research to provide even more powerful applications. It is not difficult to see that combined with big data and Internet technology, we can monitor and prevent certain infectious diseases in a timely and accurate manner, and make these activities more convenient and rapid after establishing some databases and intelligent analysis models.
2. Machine learning and blood glucose management
In November 2015, an article published in Cell magazine explained the positive significance of machine learning in nutrition. The research team first conducted a standardized diet test on 800 volunteers, collected their blood samples, feces, collected blood glucose, intestinal flora and other data, and collected questionnaires, exercise and sleep data. Researchers have found that even with the same food, different people still have very different responses. Therefore, the general dietary intake recommendations from past experience are often not perfectly matched to everyone.
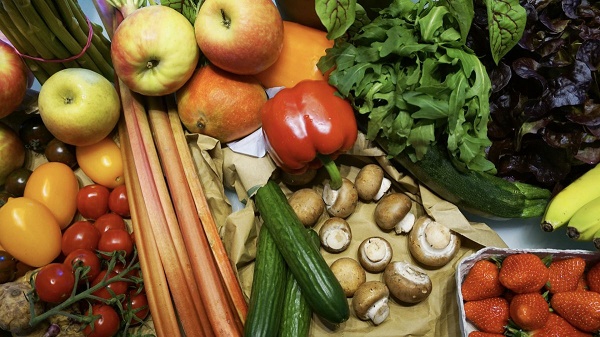
Next, the researchers developed a “machine learning” algorithm that attempts to predict the effects of blood glucose on standardized foods by analyzing the association between people’s gut microbiota characteristics and postprandial blood glucose levels. After the “training” of 800 volunteers, the predictive model established by this machine learning algorithm was validated in a new batch of volunteers.
Since then, the research team has further verified that machine learning can guide people on healthy diets. They grouped a new group of volunteers with dietary recommendations from machine learning algorithms and advice from doctors and nutrition experts. The dietary recommendations are also divided into a week of “healthy diet” and a week of “unhealthy diet”.
Through careful comparison, they found that machine learning algorithms gave more precise nutritional advice and better control of postprandial blood glucose levels, and traditional expert advice was a bit worse. It is not difficult to see that the role of machine learning has been fully reflected in this research. In precision nutrition, artificial intelligence can help users to perform accurate auxiliary analysis, so that users can make more appropriate choices.
3. Database technology and health factor monitoring
The Nuritas, Ltd. biotechnology company in Dublin is a startup that combines artificial intelligence with molecular biology. The company establishes a food database to identify whether peptides (some molecules in food products) can be used as food supplements or New ingredients. Through the use of machine learning, Nuritas can provide data mining services for food manufacturers, and plans to introduce personalized nutrition solutions for consumers in the future.
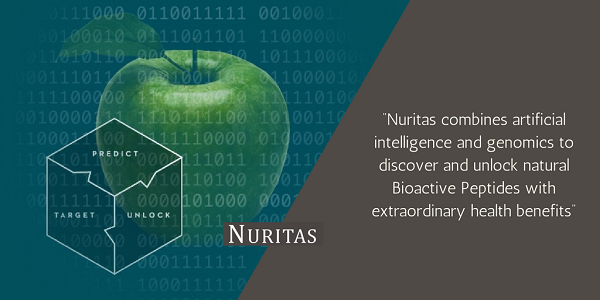
In China, artificial intelligence biotechnology startup iCarbonX is also engaged in related research and development. The company is trying to build a healthy big data platform that can ultimately be processed using artificial intelligence technology to help people manage their health. It is not difficult to see that both the food database and the health big data platform aim to monitor and record the health elements of the human body through big data and artificial intelligence technology, and through the analysis of these records and data, it is more accurate and effective. Health management plan.
4. Health management and quality of life improvement
With the continuous improvement of people’s living standards, strict management of their own health will become the daily appeal of many people. If you can collect health data from all aspects of each person, based on these data, daily management of health can be easily achieved through artificial intelligence algorithms. A considerable number of technology companies are engaged in related research. The US company Welltok is one of them.
The company’s core product is the CaféWell Health Optimization Platform (CaféWell). One of the core concepts of this product is that medical health services are not only needed by patients, but ordinary people also need to pay attention to and maintain their own health. Through technology development and service development, the CaféWell platform can help health insurers and population health managers guide and motivate users to improve their health and provide accurate health services to individuals.
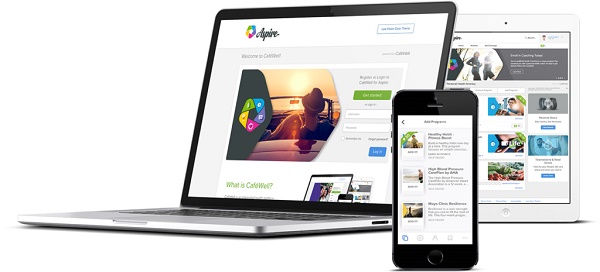
IBM also invested in WellTok and integrated its Watson platform into CaféWell, using Watson’s artificial intelligence cognitive capabilities to understand complex human languages, and quickly computing massive amounts of data to provide users with health management, chronic disease recovery and Guidance on healthy recipes, etc. Of course, other technology platforms and services similar to CaféWell are also being developed and applied. This trend stems from a higher demand for health: supplementing health services as well as health services.
5. Face recognition and emotion analysis
Emotient, Inc., a startup acquired by Apple, is committed to determining people’s emotions through facial expression analysis. Emotient originated from the University of California’s “Machine Perception Lab”, and its ultimate goal is to create a “ubiquitous” human sentiment analysis system. Emotient uses the camera to capture and record facial muscle movements, and uses its artificial intelligence calculation model to analyze facial expressions, which can interpret the meaning of facial expressions in seconds.
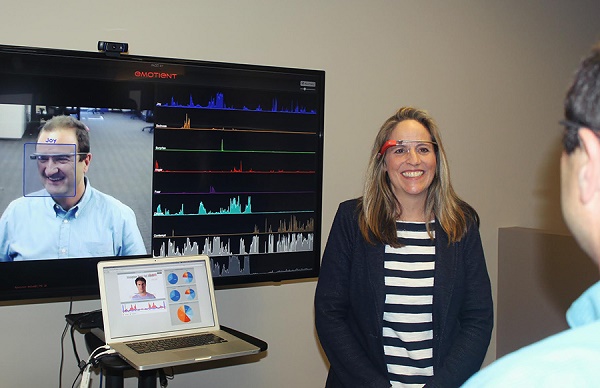
The application of this technology is actually very extensive, and when it is used in the medical field, it can be used to judge the patient’s feelings. At present, Emotient has been able to identify basic expressions such as joy, sadness, anger, surprise, etc., and can analyze some more subtle and complex expressions such as anxiety and depression. In January 2016, Apple announced the acquisition of the artificial intelligence technology company, which to some extent illustrates the potential of this technology.
6. Medical analysis and prediction of human life
People pay more attention to health in order to pursue a longer and more quality life. If you can have accurate expectations about your life, people may be able to better manage their own lives. Of course, this can also lead to some negative consequences. However, for doctors, if the patient’s life expectancy can be grasped, the patient can be better treated.
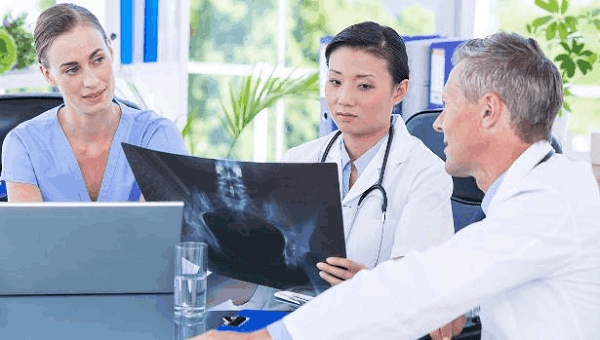
Not long ago, researchers at the University of Adelaide in Australia began using artificial intelligence to analyze medical images to predict human health and longevity. They used machine learning algorithms to analyze CT scans of 48 breasts of adults over 60 years old in the database. By analyzing these image data, artificial intelligence algorithms predict the probability of these volunteers dying within five years. By comparing with the actual situation, the accuracy of this algorithm is close to 70%, which is comparable to the prediction accuracy of medical experts.
Of course, due to the small number of research samples, the accuracy of artificial intelligence algorithm prediction has not exceeded that of human experts. However, the development of artificial intelligence relies on the expansion of data samples. If the number of patients analyzed and the number of diagnosed sites are increased, a more accurate prediction rate can be obtained, thus helping doctors to diagnose and determine treatment plans as early as possible.
Wow, amazing blog layout! How long have you been blogging for? you made blogging look easy. The overall look of your web site is magnificent, as well as the content!